I have recently joined the marketing team at Datactics and one thing I know for sure: generating accurate insights is critical for wealth and asset managers. Amongst avidly listening for soundbites during the webinar (and replaying a thousand times after to grab the best bits),
The Future Proof Operating Models webinar was always going to be an interesting one – with our very own Kieran Seaward, Head of Sales, sharing at it on the topic of Democratic Data Quality.
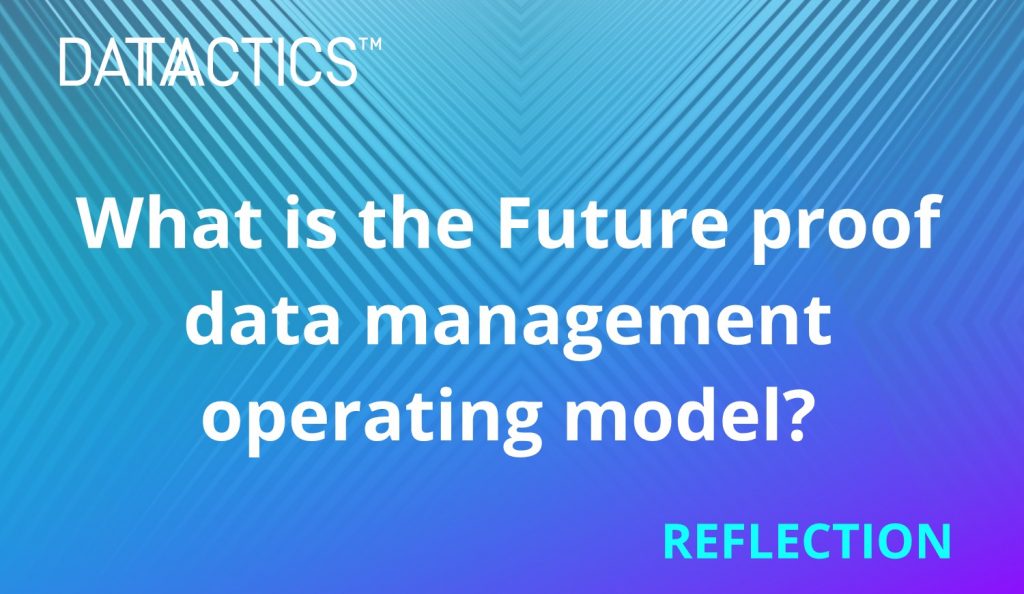
I am brand new into the data quality/ matching space and am being continually challenged with the variety and conversations that are happening. It is quite extraordinary what the software Datactics offers can achieve for companies and as I listened intently on, I thought I would take you through the journey of the webinar from start to finish…
Before diving into the weeds of data quality, Kieran provided a high-level overview of the standard building blocks of data management and helpfully positioned stated where data quality sat and how it adds value to the overall data estate. After explaining data governance tools highlighting Collibra and Informatica for reference he considered data lineage systems and how they use the metadata ‘stamp’ to track data as it flows throughout the organisation.
Why?
This enables users to comprehend where the data originated from and who has made changes to data at any point and time. He went to explain the importance of master data quality management and how it is the repository of the ‘golden copy’ of all your business-critical data.
Having provided this context, data quality management was discussed. DQM actively ensures data is fit for consumption and that efficiently meets the needs of data consumers.
Data must be consistent and unambiguous to qualify as of a high standard. Data Quality can be measured to ensure industry-standard dimensions, starting with the most basic: completeness.
One major takeaway of the webinar was the explanation of the root causes of poor data quality. These issues are often traced back to duplication caused by database merges, format inconsistencies; or furthermore, human error. An interesting parallel that Kieran made was that data that is not high quality should not only be identified and cleansed but also analysed.
Why?
To identify the root cause and preventative measures put in place to improve data quality over time. What is this approach referred to? A ‘bottom-up’ approach to data management.
And finally, Data Quality management ensures data is fit for consumption and meets the needs of data consumers.
To be of high quality, data must be consistent and unambiguous.
You can measure data quality to industry-standard dimensions, starting with the most basic; completeness i.e. is data present; conformity – does it conform to a particular format; to the more complex: accuracy and uniqueness.
The root causes of data quality issues are often traced back to: duplication caused by database merges, format inconsistencies or human error.
Data that is not high quality should not only be identified and cleansed but also analysed to identify the root cause and preventative measures put in place to improve data quality over time. This is sometimes referred to as the ‘bottom-up’ approach to data management.
What is currently happening with the traditional approach to data quality?
Well, as Kieran stated business units should stand up to data quality solutions.
For example, the marketing department would require DQ rules to improve customer analytics. A Chief Operating Officer would want to assign data quality ‘breaks’ to stewards who are responsible for the data to enable them to fix it.
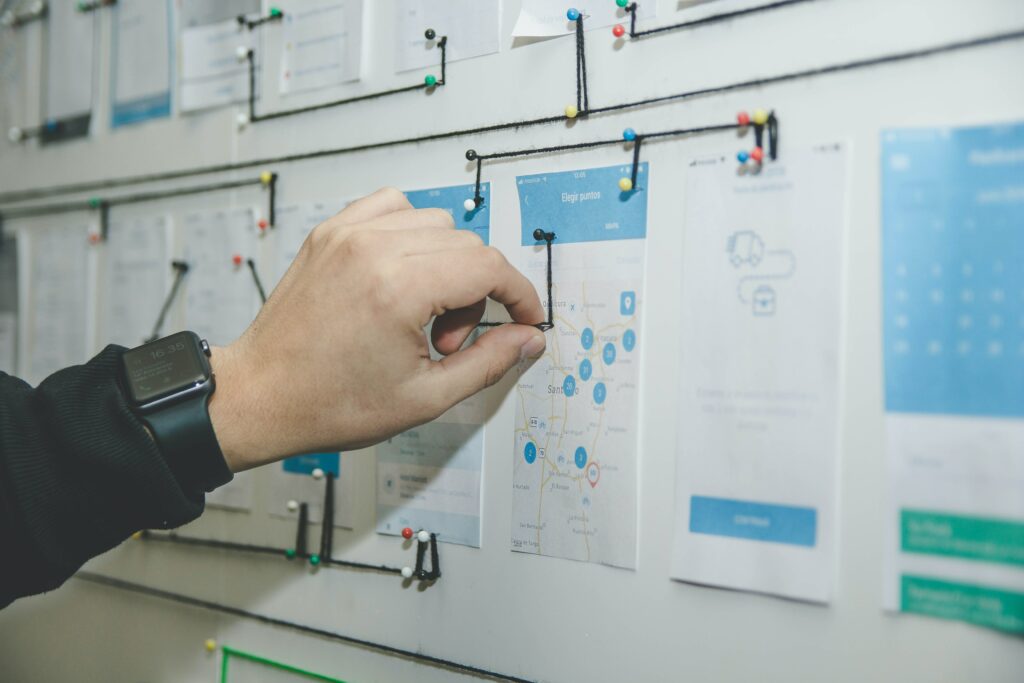
Another example would be Risk or Reg reporting teams who need to measure the data quality of reports before sending them to the regulator. As a result of this, traditional data quality models can hamper the process due to the requirement of program resources to hard code rules.
IT departments are often stretched as is, and they do not have the resources available to meet the demands of the business, therefore they end up with a large volume of requests to provide ready to use data and not the resources to deliver this.
With this in mind, Kieran pointed out that the turnaround time to stand-up Data Quality solutions is much too long – meaning something has got to change.
Kieran went on to explain what the Future proof data management operating model is. But what exactly is it that makes a data management operating model future proof? Well, banking firms have increasingly been moving the responsibility of data quality away from IT departments and have been seeking to empower business users who know and use the data (seeking to give them the ability to make decisions and fix that data).
These data stewards are arguably the most knowledgeable on subject matters.
With increased demands on business (likely will increase particularly post COVID 19), rather than relying on IT to code/program rules, the data management office can be enabled to provide an internal data quality service to the business.
How can this be done?
Kieran explained that the tools need to match the profile of the user. Future operating models need to be agile and flexible to enable connection with existing systems and allow businesses to gain competitive advantage.
“Utilising the off the shelf tooling will assist you to ensure both centralised and standardised data quality operations without ripping and replacing infrastructure”.
The golden nugget of the webinar was that you don’t need to wait for the next modern digital technologies and services upgrade to be the answer to all your problems. You have the option to measure and improve data quality TODAY.
At this point, Kieran proceeded to educate on the features of the model. With the model seeking to enable users to affect long term cultural change, it is key to make it as easy as possible to effectively fit every user.
How?
Well, firstly through rule creation – this is going to be performed by data management/ data governance analysts so, therefore, the products and services needs to not require programming or coding to update rules. Many of these rules will be reusable from one solution to the next.
How will the model impact the day to day role of the user?
It won’t – the operating model includes minimal impact on the user’s everyday role.
The visualisation of summary data quality statistics in an interactive dashboard helps to provide business users with a clear visual understanding of the health of data within their domain.
Kieran further clarified that business stewards perform a very important function in this model – the performance of root cause analysis and remediation of data quality issues.
How can this be achieved?
This can be achieved on a record by record basis – Artificial Intelligence and Machine Learning techniques can, however, be used to reduce the volume of manual effort required for data analytics.
What is the result?
This will then feedback over time into a continuous loop that will see incremental improvement in data quality over time. Lastly, the system requires smart workflow automation.
What does this mean?
Well, the system needs to connect to many different systems of information across the business therefore it requires smart workflow automation and a range of connectivity options ‘out of the box’.
What does this enable?
The component enables users to schedule automatic runs of the solutions and handle all the associated outputs.
At this point in the session, the best part was revealed: THE BENEFITS.
We have heard about the traditional approach to data quality (and the subsequent gaps), we have heard about what the future proof data management operating model is, and we have heard what the features are of the target operating model.
Now, we will consider the benefits. Kieran succinctly explained what the benefits the clients are experiencing with this new business model.
Firstly, the ability to democratise data quality, as explained earlier this will empower the people who know the data (the subject matter experts) to fix the data.
Secondly, the ability to access control, this will help with privacy to ensure that only the individuals with permission have accessibility to the data (particularly integral for wealth management firms). Kieran explained for reference that Datactics have used Microsoft Active Directory to understand which users are entitled to access different data types.
Thirdly, from a marketing perspective, customer intelligence is offered. The model allows a greater, more in-depth understanding of your customers with improved data quality as a result.
What about data migration?
The model is perfect for wealth managers who require to be in a strong position considering an acquisition occurring meaning data needs to be migrated/ consolidated. From a perspective of the present day, the rapid response the model provides will be hugely advantageous particularly when facing unprecedented times such as COVID 19.
Kieran finished the session off with a client example of Datactics which helped to solidify what he has said.
Amid COVID 19, a client considered the scenario of, ‘how can we maintain customer relationships if we have no postal service available?’. This question was posed to the data management team (users of Datactics). We all know that email addresses are crucial to customer relationships, they not only share documentation and product updates, but they provide opportunities for companies to upsell new products.
Kieran carried on to state that the client asked ‘how many of the email addresses are missing from the contact system? How many email addresses are in the right format?’. Well, the Datactics platform was able to build a solution in under 2 hours that was able to give the client an insight into the proportion of relevant emails that were in the correct format.
This example presented by Kieran illustrated that a business-driven problem like this can be assisted by Datactics tools. Furthering this, the data management team was able to ‘self serve’ entirely for this requirement (which has gone down well). Kieran ended the webinar session by reiterating that the contact is hopeful that non-data experts in senior management roles will be able to comprehend the business relevance that data quality improvement can have for business-critical activities. The end of the session saw questions answered eloquently by Kieran.
Overall, the webinar was great. From start to finish the building blocks of data quality management were laid out, which then saw the discussion of traditional approaches to data quality management. Furthering this, the future proof data management operating model was introduced with usage, feature, and benefits explored. Kieran concluded with a Datactics client example which helped to indicate the requirement for our data quality tools in COVID 19 and in every other unprecedented that may arise. By pinpointing the features and benefits, it was clear that the solution was not only sound but solving a real problem. This webinar was highly interesting and as a new start in this mad world of data – it is clear to see that the masses of data that exist need to be carefully managed and furthering this the subject matters (business users) being able to understand the data is massively advantageous. At the end of the day, empowering business users who know and use the data is critical.
Click here for more by the author, or find us on Linkedin, Twitter or Facebook for the latest news.