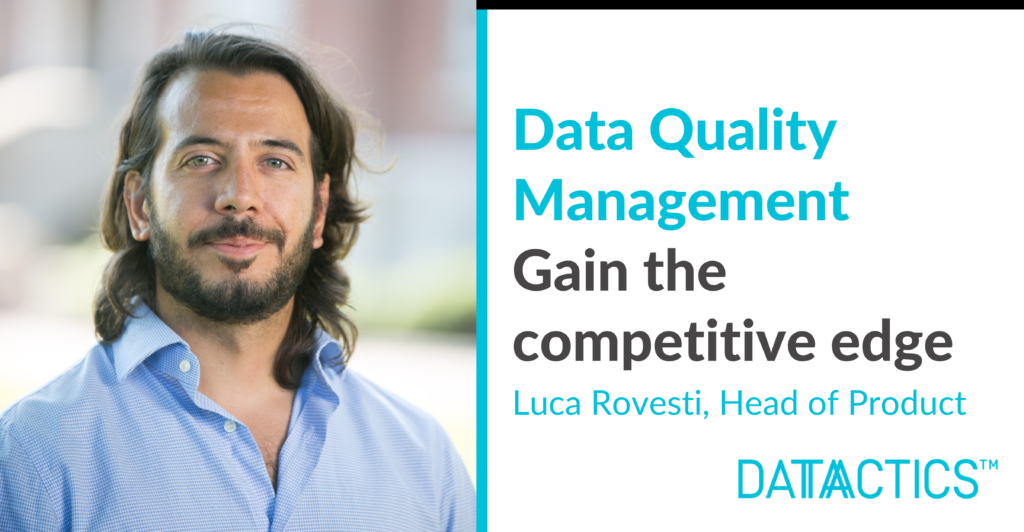
Managing data assets has become a way to gain competitive advantage for businesses and is a keen focus area for regulatory bodies around the globe. With the emergence of data standards and a plethora of technology businesses, the data management landscape has never been so competitive.
The following blog will look at how to maximise your data assets and gain that competitive edge by developing a data quality management framework, making use of best-of-breed technologies.
Tech solutions for data quality management
Back in the 16th century Sir Francis Bacon wrote that “knowledge itself is power”, conveying the idea that having knowledge is the foundation of influence, reputation, and decision-making. In modern times, the information we use to acquire knowledge is highly digitalised, often in a collection of organised data points. Every individual and every organisation in the world is faced with the challenge of managing data. The bigger the organisation, typically the more complex the challenges are in managing data used to make business decisions.
How to gain the competitive edge in such fast-evolving industry? Arguably, a mix of technologies and process accelerators aimed at solving the right problems.
The goal of best-of-breed-technology should be to automate manual, time consuming tasks required to govern enterprise data (such as data profiling and data cleansing), whilst streamlining anything that is not possible or desirable to automate. The automation challenge can be solved by deploying supervised machine learning solutions, which should be functional to solving problems identified in the data governance framework and should adhere to data stewardship and data security principles. Explainable AI with person-in-the-loop feedback and decisions is a sensible way to achieve this, as users can understand the rationale behind a model’s prediction.
In terms of Data Quality, the aim is to provide a self-driving solution that can cope with sensitive data in big volumes and at scale. In order to gain a competitive edge, it should be interconnected with other players in the data management ecosystems such as governance, lineage and catalogue platforms. In order to add value to an organisation’s data assets, data stewards from all data domains should feel empowered to get on top of quality issues and make use of powerful tools to perform remediation.
Let’s explore some of the conceptual synergies that exist between actors in the data management ecosystem. A data governance framework is the theoretical view of how data should be managed. Integration with data quality and lineage provides the practical evidence of guidelines being followed or overlooked.
Data ownership is a fundamental aspect driven by governance, the definition of which is a must-have success criterion for any data quality initiative. Governance and data catalogues also set the glossary and terminology standards to be used across the data management tools.
Looking at data quality and lineage, these two players are complementary in their ability to track data records in motion, from data source to output. Quality of the data ultimately means acknowledging that it is ‘fit-for-purpose’ and lineage tools are powerful in visualising the ramifications of where data originally comes from and what they are being used for. Data quality in motion is compelling as it looks at how the data flows through your organisation; identifying where problems originate from (root cause analysis) and it also gives a clear picture of the ultimate impact of DQ issues.
Why we do data quality
We’re now going to shift the focus from “how we do data quality” on to “why we do data quality”. In today’s marketplace, technology vendors are always faced with the challenge of quantifying their value proposition. In other words, quantifying the “cost of data quality”.
It is a complex function made up of multiple variable, but here is an attempt at summarising the key concept. Whilst good quality data has the potential to improve business operations and outcomes, poor data quality is capable of hindering this (or worse). Below are a number of potential outcomes if bad data is allowed to manifest within an organisation:
- Regulatory risks. Depending on the industry, there will often be a considerable risk in terms of monetary fines and/or operational restrictions depending on the severity of the data quality issues. Importantly, the risks are not only associated to the actual data problems, but also to the lack of processes. For example, having a data governance program in place to identify and rectify potential data problems is invaluable to a financial institution battling with regulation and compliance, in order to avoid costly fines (e.g. GDPR).
- Opportunity cost. This refers to the ability (or inability) to make use of information in order to gain competitive advantage. For example, this could refer to the reduced ability to cross-sell products to the customer base because of missing connections between customer and product data. Sub-optimal data assets can therefore have costly repercussions for a business losing out on available opportunities.
- Operational risk. If a business or organisation continues to work with poor quality data, there is a chance it could fall victim to operational risks. The failure of identifying a risk factor such as fraudulent behaviour and/or conflicts of interest could be due to a lack of data integration, missing links or unclear hierarchies in the data. This is a typical focus area for Anti-Money-Laundry and KYC projects.
- Severity of data quality issues. This simply refers to the capability of a data owner to understand the business impact of a data quality issue to an operation. This is in itself is a difficult variable to estimate, for it needs to take into consideration the context around the faulty information and the criticality (size) of the problem for the operation. To give a practical example, put plainly, a data quality issue associated with a large investment is more severe than the very same issue associated with a smaller investment.
Overall, the ability to gain a competitive edge will hinge on the existence of a mix of technological and process-driven innovation (=how we do it), correctly focused on addressing the key problems in data management (=why we do it!). There are plenty of business benefits from creating a data governance framework and mastering data quality, from less risk potential, to greater business opportunities and improved operations.
If you are developing a data management framework or are seeking to understand our data quality solution, you can read more about it in our datablog. Similarly, if you would like to speak directly to us at Datactics, you can reach out to Luca Rovesti.