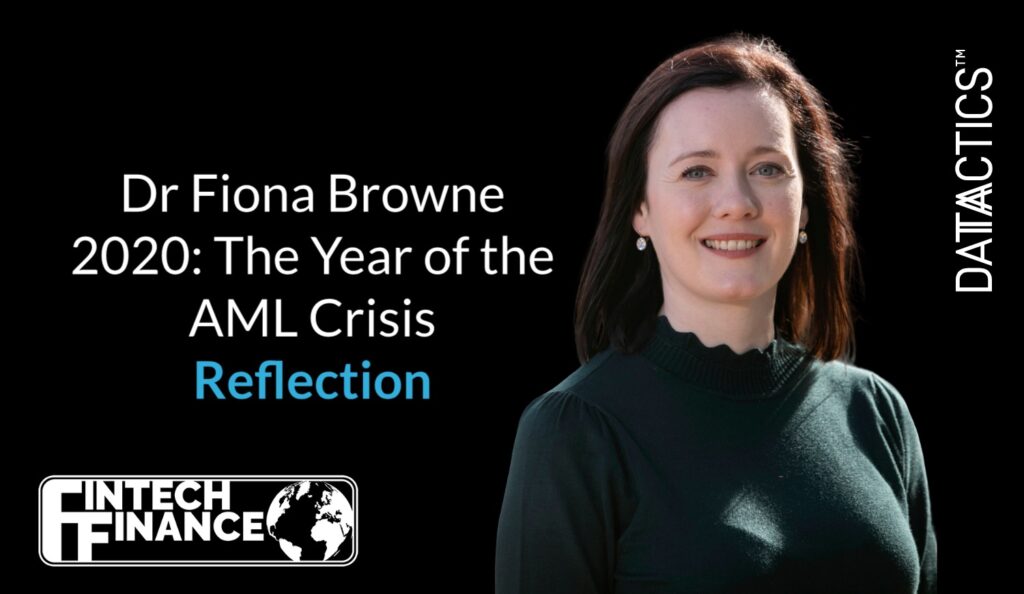
Datactics Head of Artificial Intelligence, Dr. Fiona Browne, recently contributed to the episode of FinTech Finance: Virtual Arena. Steered by Douglas MacKenzie, the interview covered the extent of the Anti-Money Laundering (AML) fines currently faced by banks over the last number of years and start to unpack what we do at Datactics in relation to this topic: helping banks address their data quality, with essential solutions designed to combat fraudsters and money launderers.
How can banks arm themselves against increasing regulatory and technological complexity?
Fiona began by highlighting how Financial Institutions face significant challenges when managing their data. However, the increase in financial regulations since the financial crisis of 2008/2009, ensuring data quality has gained in its importance, obliging institutions to have a handle on their data and make sure it is up to date. Modern data quality platforms mean that the timeliness of data can now be checked via a ‘pulse check’ to ensure that it can be used in further downstream processes and that it meets regulations.
Where does Datactics fit in to the AML arena?
A financial institution needs to be able to verify the client that they are working with when going through the AML checks. The AML process itself is vast but at Datactics, we focus on the area of profiling data quality and matching – it is our bread and butter. Fiona stressed the importance of internal checks as well as public entity data, such as sanction and watch lists.
In a nutshell, there is a significant amount of data to check and compare and with lack ofquality data, it becomes a difficult and costly task to perform so we at Datactics, focus on data quality cleansing and matching at scale.
Why should banks look to partner, rather than building it in house?
One of the key issues of doing this in house is not having the necessary resources to perform the required checks and adhere to the different processes in the AML pipeline. According to the Financial Conduct Authority (FCA), in-house checks and a lack of data are causing leading financial institutions to receive hefty fines. Fiona reiterated that when Banks bring it back to the fundamentals and get their processes right and data into order, they can then use the partner’s technology to automate and streamline these processes, which in turn speeds up the onboarding process and ensure the legislation is being met.
Why did the period of 2018/2019 have such a high number of AML breaches?
Fiona explained that many transactions go back over a decade, it takes time to identify such transactions. AML compliance is difficult to achieve and regulators know that it is challenging. The regulators are doing a better job at providing guidelines to financial institutions, enabling them to address these regulations. Fiona reaffirmed that perhaps 2018/2019 was a wakeup call that was well needed to address this issue.
And with AML fines already at $5.6 billion this year, more than the whole of 2019, what can banks do?
Looking at the US, where although the fines for non-compliant AML processes are not as high as 2019, there is still a substantial number of fines being issued, Fiona said that it is paramount to ensure financial institutions have the right data and the right processes in place. Although it can be considered as an administrative burden, there is real criminal activity behind the scenes, which is why AML is so important. It is vital that financial institutions get a handle on this, enabling them to also improve the experience for their clients.
The fines will continue to be issued. Why should firms look to clean data when they just want to get to the bottom line?
It is essential to have the building blocks in place. Data quality is key for the onboarding process, but it is also essential downstream, particularly if you are wanting to do more trend analysis. Getting the fundamentals right at the start will pay back in dividends.
Are there any other influences that Artificial Intelligence (AI) and Machine Learning (ML) can have on the banks onboarding process?
According to Fiona, there is no silver bullet. One AI/ML technique will not solve all the AML issues. It is about deploying these techniques when approaching the issues in different ways. A large part of the onboarding process is gathering data and extracting relevant information from the data set. Fiona has seen a lot of Neuro-Linguistic Programming (NLP) techniques employed to extract the data from documents. At Datactics, we use Machine Learning in the data matching process to reduce the manual review time. ML techniques are employed in supervised and unsupervised approaches geared to pinpoint fraudulent transactions. We think that the graph databases and network analysis side of machine learning is an interesting area, we are currently exploring how it can be deployed into AML and fraud detection.
Bonus content: In the US and Canada, one way to potentially identity fraud was to look at transactions that were over $10,000. The criminals however become increasingly savvy and utilise Machine Learning to muddy their tracks. By doing this, they can divide transactions into randomised amounts to make them appear less pertinent. As Fiona put it ‘the cat and mouse game’.
If you are employed in the banking sector or if you must deal with large and messy datasets, you will probably face challenges derived from poor data quality, standardization, and siloed information.
Datactics provides the tools to tackle these issues with minimum IT overhead, in a powerful and agile way. Get in touch with the self-service data quality experts today to find out how we can help.
You can also watch our AML screening in action here or read our latest whitepapers.