How to achieve Data Quality for your Data Fabric
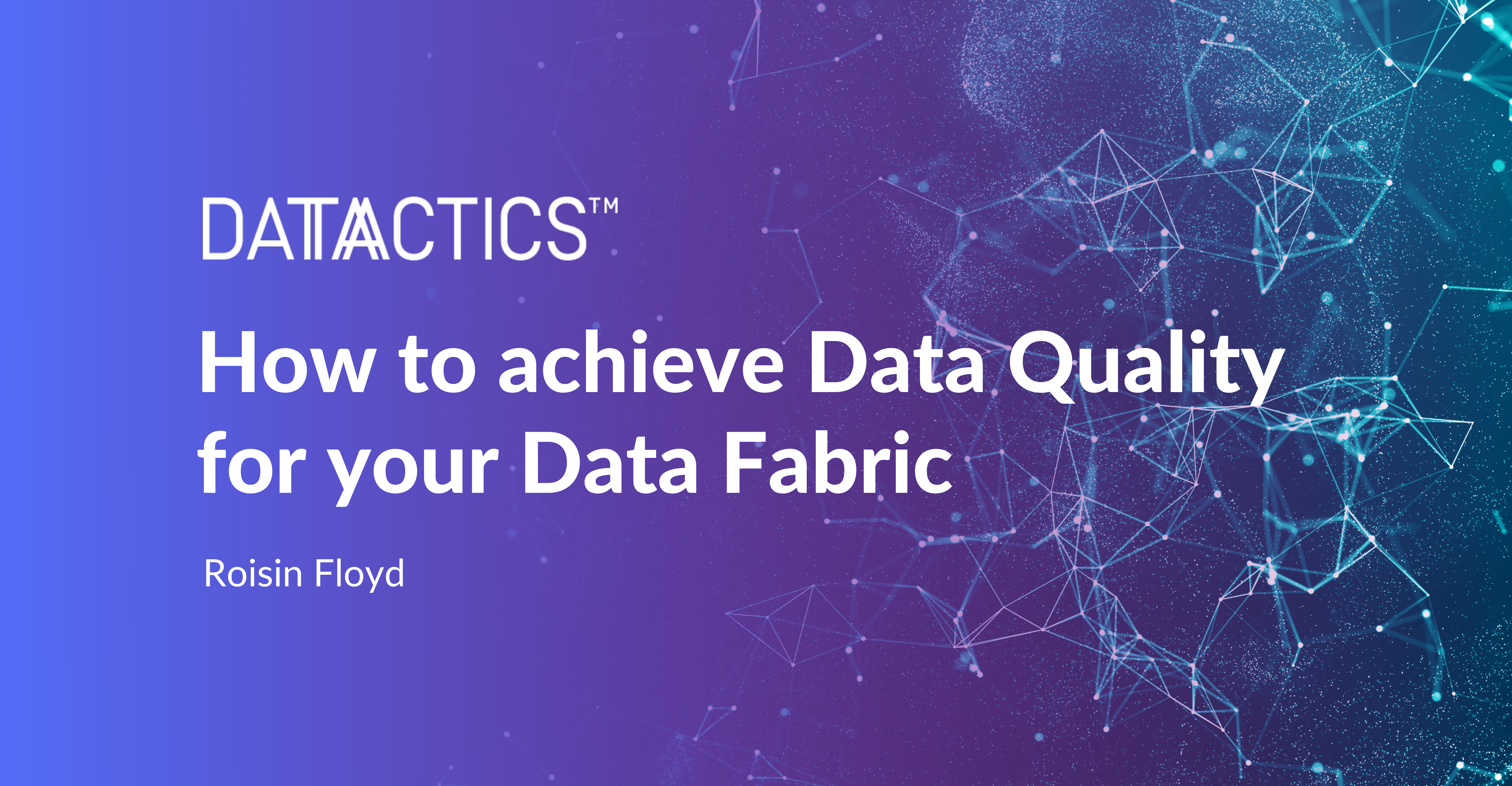
In 5 years, it is predicted that most organisations that are data driven and serious about digitisation will have created a data fabric and associated tooling. This resource provides clean information into business processes which can power critical solutions from creating single customer view or regulatory reporting to predictive analytic models for better decision making in your company’s operations.
“By 2024, data fabric deployments will quadruple efficiency in data utilization, while cutting human-driven data management tasks in half.”
Gartner®, Emerging Technologies: Critical Insights on Data Fabric, 2022
But what many people don’t realise is that the success of a data fabric design hinges on the quality of the data within it. Without accurate and trustworthy data, decision makers can’t make informed decisions and business processes can’t function as intended. In this post, we’ll explain why good data quality matters to data fabric and offer some tips for ensuring that your data is up to par.
How does it work?
A data fabric is an architecture that enables data sharing and exchange across a distributed data environment, combining key data management processes such as data governance and data catalog. It allows organisations to connect data from disparate data sources, process and analyse data in real-time, and provide employees with access to the data they need to make better decisions. Gartner offers this simple explanation for how data fabric works,
“Data fabric is an emerging data management design for attaining flexible, reusable and augmented data management (i.e. better semantics, integration and organization of data) through metadata. Metadata drives the fabric design. Compared to traditional approaches, active metadata and semantic inference are key new aspects of a data fabric.
Data fabric is NOT a rip and replacement of existing data management infrastructure. The fabric design evolves over time. Initially, existing systems can passively participate in the fabric design by sharing their metadata. When the fabric design matures, participating systems actively adapt to the alerts and recommendations generated by the fabric through data and AI orchestration.
A data fabric can be designed and deployed manually or augmented using automation techniques. As perpetual manual efforts are unsustainable, and a fully automated data fabric is unachievable, a practical data fabric ONLY covers the best of both.”
What are the benefits of a data fabric?
Alongside improving trust in the data among data consumers, the business value of data fabric implementation can be significant. Gartner comments that “Metadata-driven data fabric has significant business value potential to reduce data management efforts, including design, deployment and operations (see Top Trends in Data and Analytics, 2022)”.
In other words, a data fabric implementation can offer a multitude of benefits across your organisation’s functions. Implementing a data fabric can help you to better manage and govern your data, ensuring that it is compliant with regulations. It also helps you to achieve a single, unified view of your data, making it easier to gain insights that were previously siloes. It can also help to quickly and easily share data across different departments and organisations.
Your key ingredient to a successful data fabric implementation
Data fabric implementation can be challenging due to the need to manage data quality, security, and governance across a distributed data landscape. In addition, data fabric design can be complex, requiring extensive planning and coordination to ensure all data stakeholders are able to work together effectively. But most crucially, data fabric implementation relies heavily on good quality data in order to get the most out of its architecture.
Data quality is the key ingredient for implementing a successful data fabric architecture into your organisation. Data quality, whilst important to have in good condition for any data management programme, is critical for a data fabric implementation for a number of reasons.
First, data quality affects the overall performance of the data fabric. Poor data quality can lead to data inconsistencies and data loss, which can in turn lead to reduced performance and decreased data availability.
Second, data quality plays a role in data security. In order to maintain data security, data must be accurate and complete. Data that is inaccurate or incomplete is more vulnerable to being accessed by unauthorized individuals.
Third, according to Gartner, “compatible metadata and assured data quality are essential to joining data across the enterprise into common models like ontologies. Once data is mapped to a business ontology, the business gets a good set of connected data models that are ready for analysis.”
How to address data quality challenges
Data quality challenges can arise from a variety of sources, including errors in data sets, data cleansing errors, and data transformation errors. To address these challenges, organizations need to put in place processes and controls to ensure that data quality is maintained at a high level throughout the data fabric implementation process. By doing so, they can ensure that their data fabric implementations are as effective and efficient as possible.
There are a few ways of measuring data quality (and in turn generating better data integrity) using the following data quality dimensions: Accuracy – This means that data should be free of errors and accurate. Completeness – Meaning all data points should be included and no data should be missing. Consistency – This means data should be consistent across different data sources. Timeliness – Data should be up-to-date. By taking some time to carry out data preparation and making sure data is of good quality before it is inputted into the data fabric, the data fabric will work more effectively.
There are a few things that can help ensure data quality: Data cleansing – This involves identifying and correcting errors in data. Data transformation – This involves converting data into a format that is easier to work with and read. Data standardization – This involves setting standards for how data should be inputted and formatted. By taking these steps to ensure data quality, the data fabric will be more effective.
Data quality software can help to create a data fabric that is fit for purpose. By automating data quality processes, organisations can improve the accuracy of their data and free up resources to focus on other areas. Data quality software can also help to identify and correct errors in data, preventing incorrect decisions from being made. As a result, data quality software can play a vital role in helping organisations to transform their data quality and gain insights that can help them improve their business operations.
To conclude…
The consequences of poor data quality are not only felt in the accuracy of analytics and reporting, but also in the overall performance of the data fabric itself. That’s why it’s so important to use data quality software as part of your fabric implementation. Data Quality Software automates many of the processes needed to ensure high-quality data, such as cleansing, standardization, and enrichment. This allows you to focus on other aspects of your fabric deployment while still ensuring that your data is ready for analysis.
If you want to learn more about how data quality software can help with your data fabric implementation, reach out to us via the button below. We would be happy to answer any questions you have and help you get started.
Gartner, Quick Answer: What Is Data Fabric Design?, Robert Thanaraj, Mark Beyer, Ehtisham Zaidi, Guido De Simoni, 28 March 2022
GARTNER is a registered trademark and service mark of Gartner, Inc. and/or its affiliates in the U.S. and internationally and is used herein with permission. All rights reserved.