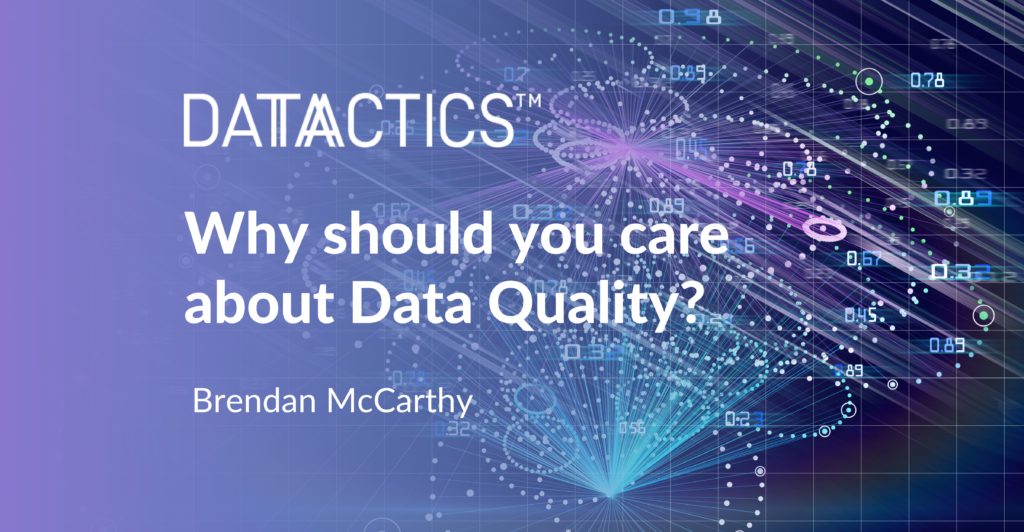
Data quality may not be viewed as a particularly attractive topic of conversation at a dinner party or a company event, yet it is a critical step for organisations to maximise the value of their information and subsequent decision-making. It is therefore imperative that data leaders make every attempt to raise awareness and educate on why data quality is so important to an enterprise’s success – and ultimately make people care about data quality.
To begin, what exactly is meant to by data quality?
High quality data is defined as data that is fit for purpose, reliable and trustworthy. While organisations may maintain different quality standards, the Data Management Association UK (DAMA) propose that in order to be considered high quality, data must satisfy the following six dimensions; accuracy, completeness, uniqueness, consistency, timeliness and validity. These are often viewed as the foundations of data quality, however it can be argued that technical measurements and standards are determined by the use case.
Data problems can destroy business value. Recent research from Gartner shows that organisations estimate the average cost of poor data quality at over $10 million per year, a figure which will likely increase as the modern business environment becomes increasingly digitalized and unstructured data becomes harder to decipher. It’s also estimated that in 2021, eight out of ten companies admitted to struggling with data quality issues.
Senior leadership often neglect data quality as an organizational priority unless they are provided with an immediate reason to address the issue. Only when bad data quality is demonstrably proven to have a negative impact on the business will action be taken; bad data that casues complications to the initiatives and processes that senior management care about and are essential to the business.
Data quality lays at the foundation of all data management, data governance and data lineage processes; therefore it is imperative to get it right and ensure your business embraces a culture that cares about data quality.
In order to get your organization talking about the importance of good data quality, here are some recommendations:
First step: directly expose the pain caused to business operations by bad data quality;
- This can be communicated to senior leaders and stakeholders through presenting a problem statement or a business case which they can own and align with the current strategic objectives of the firm. Connecting the business case with the organizational trajectory will help senior leaders understand the operational and financial benefits of addressing data quality issues. Also, this can help you answer the inevitable “why do we need to do this?” question.
- Business leaders can also be engaged with end users to discuss their experiences, share anecdotes and help senior management emotionally connect with those at the firm who are regularly impacted by the consequences of poor quality data.
- It may also be of merit to present historical events where firms have suffered from bad data quality, resulting in organizational disasters or heavy fines from financial regulators.
Completing these actions will help focus your business case on improving the health and quality of data that matters to the stakeholders who care about the problem and are prepared to help instigate an institutional change in organizational attitude’s to data quality.
Second step: Shift the company culture to one that cares about data quality management. Present key metrics which illustrate the tangible impacts of poor data quality to the organization and outline the resources that are required to make a change;
- The key processes and process owners (needed to deliver on outcomes) must be identified and will likely span across multiple business areas. This will alleviate any concerns on siloed thinking between functional areas and elevate the role that data quality plays across the firm.
- Work with key process owners to determine the key indicators which will be most critical to the newly identified business processes. This will help you define your critical data elements and the data quality associated with them e.g. quality of Customer Contact master data.
- Data profiling and analysis of critical data elements can help to demonstrate their impact on business performance. This can be carried out via in-house or external data management tools, or using programming scripts such as Python or SQL. Results must be communicated and explained to business stakeholders to infer how data quality limitations on critical data elements can hinder organizational performance and how improvements can contribute to superior results.
- Identify alternative areas of the business where the importance of good data quality is imperative as the business scales e.g. data science, advanced analytics and machine learning. Good data quality is fundamental to generate business value from these areas and therefore must be addressed.
By completing these two steps, your key business stakeholders will have developed an empathetic and rational understanding of the day to day operational benefits of data quality improvement. By creating an organizational culture that cares about data quality, business users will hopefully see data quality issues propelled to the forefront of the firm’s IT and data management strategy. Ideally, this may lead to additional funding and available resources to tackle data quality issues.
To have further conversations about the drivers and benefits of a Self-Service Data Quality platform, reach out to Brendan McCarthy.
And for more from Datactics, find us on Linkedin, Twitter, or Facebook.