Despite a seemingly healthy green glow in your dashboards and exemplary regulatory reports, you can’t help but sense that something is amiss with the data. If this feeling rings true for you, don’t worry – it may be an indication of bigger issues lurking beneath the surface.
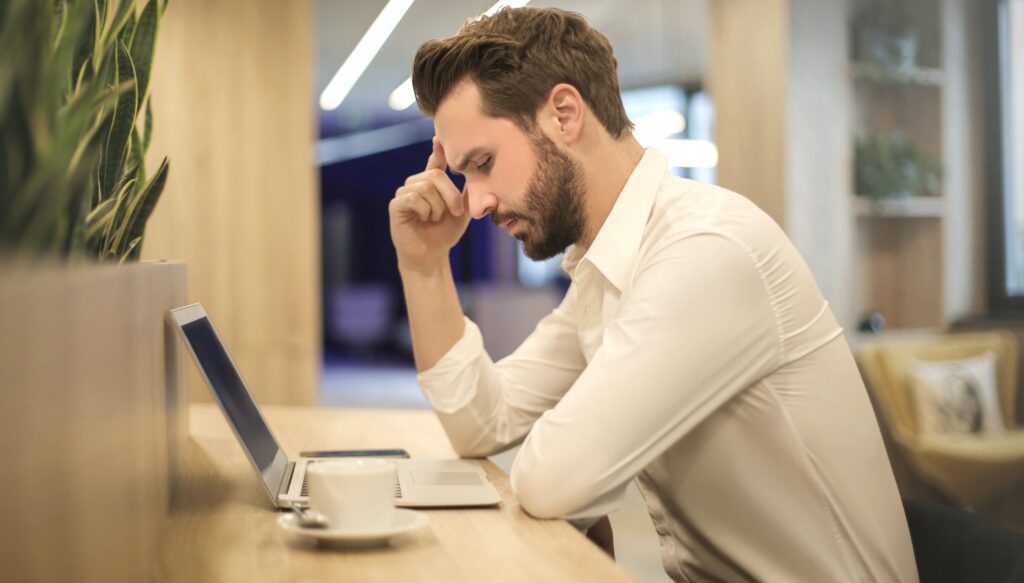
You’re not alone. In this blog we’ve taken a look at some of the most influential factors that indicate you’ve got a data quality problem. Why not use these handy pointers as a starting point to dig deeper?
1. You’re getting negative feedback from your internal business partners.
Data is the backbone of any business, so it’s no surprise that a lack of satisfaction from internal partners can often be traced back to data issues. From ensuring quality datasets are delivered at scale, through to solutions aimed towards empowering your colleagues with access to necessary information and context – there are many proactive steps you can take when aiming for better performance in this area. Taking action now will ensure everyone has what they need; fuelling success and transforming negative feedback into positive progress.
2. People keep sending you data in Microsoft Excel.
Now, we all love Excel. It’s brilliant. It’s made data handling a far more widespread expectation at every level of an organisation. But it does not give any way of source or version controlling your datasets, and is massively prone to its inherent limitations in scale and size. In fact, its ubiquity and almost unilateral adoption means that all your fabulous data lake investments are being totally undermined when things like remediation files, or reports, get downloaded into an Excel sheet. If you’re seeing Excel being used for these kinds of activities, you can bet you’ve a data quality problem (or multiple problems) that are having a real effect on your business.
3. Your IT team has more tickets than an abandoned car.
If your business teams aren’t getting the data they need, they’re going to keep logging tickets for it. It’s likely these tickets will include:
- Change requests, to get the specific things they need;
- Service requests, for a dataset or sets;
- Issue logs because the data is wrong.
More than an identifier that the data’s not great, this actually shows that the responsibility for accessing and using the data remains in the wrong place. It’s like they’re going to a library with an idea of the plot of the story, and the genre, but they can’t actually search by those terms so they’re stuck in a cycle of guessing, of trial and error.
4. Conclusion
What these indicators have shown is that identifying data quality issues isn’t just for data teams or data observability tools to own. The ablity to recognise that something isn’t right is something that sits just as importantly within business lines, users and teams.
What to do next is always the key question. Ultimately, data quality can be improved if the right processes and tools are put in place to collect, cleanse, and enrich data. There are several challenges that need to be overcome when dealing with bad data. These challenges include:
- Identifying data quality issues,
- Deploying adequate resources and time to resolve them, and
- Investing in advanced analytical tools.
To do this effectively, enterprise-wide data governance is essential as it provides an actionable framework for businesses to continuously manage their data quality over time. Although implementing changes across an organisation may seem daunting at first, there are a few simple steps which organisations can take today that will help them quickly improve their grip on data quality.
A very important first step is the establishment of a data quality control framework, and helpfully we’ve written about this in the following blog. Happy reading!