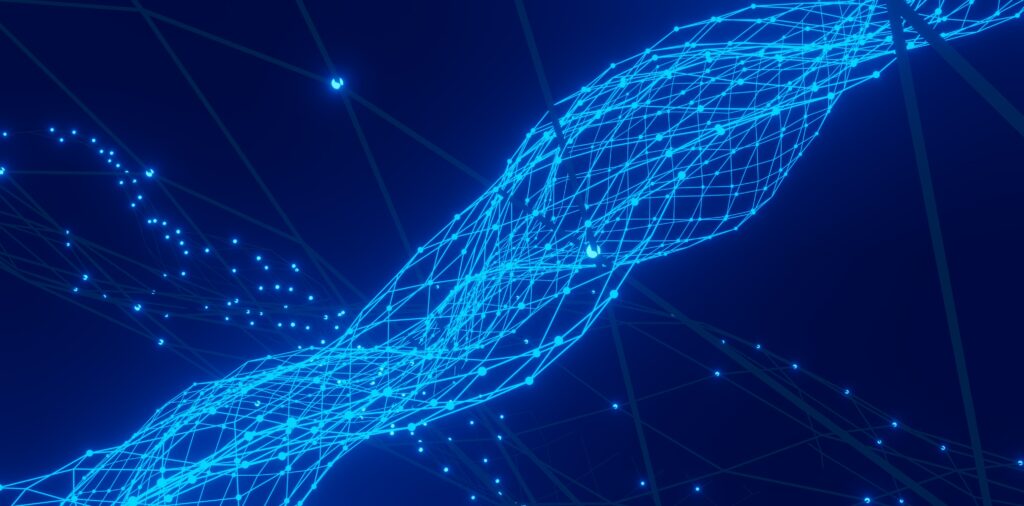
If you consider data as the lifeblood of your organization, trying to manage it in a static, distributed fashion seems like a challenging and almost futile exercise. Adopting a data fabric or mesh approach is important as it enables better management of data in-motion as it flows throughout the organization. Moreover, it allows for potential to add value through a greater variety of use cases.
Any organisation which values their data as an asset would benefit from a holistic approach to data management. By considering a data fabric implementation, businesses can unlock a more efficient, secure and modernised approach to data analysis and management.
What is a data fabric, and how does it differ from a data mesh?
Data fabric has been defined by Gartner as,
“…a design concept that serves as an integrated layer (fabric) of data and connecting processes. A data fabric utilizes continuous analytics over existing, discoverable and inferenced metadata assets to support the design, deployment and utilization of integrated and reusable data across all environments, including hybrid and multi-cloud platforms.”
Gartner, 2021
Specifically, Gartner’s concept means that both human and machine capabilities can be leveraged so that data can be accessed where it resides.
It is a term coined by Noel Yuhanna of Forrester, whereas Mesh was noted down by Zhamak Dehghani of a North American tech incubator, Thoughtworks. Essentially they’re two similar but notably different ways of expressing how firms are approaching, or should approach, their data architecture and data management estate, usually comprising bought and built tools for data governance, data quality, data integration, data lineage and so on.
Both approaches describe ways of solving the problem of managing data in a diverse, often federated and distributed environment or range of environments. If this seems like a very conceptual problem, perhaps a simpler way is to say that they are ways of providing access to data across multiple technologies and platforms.
In the case of a data fabric, it can assist with the migration, transformation and consolidation required to coalesce data where this meets the business need (for example, in migrating to a data lake, or to a cloud environment, or as part of a digital transformation programme). In its thorough research piece, targeted at data and analytics leaders exploring the opportunity to modernise their data management and integration approach, Gartner has detailed some benefits in a theoretical case study based on a supply chain leader utilising a data fabric:
“…(they) can add newly encountered data assets to known relationships between supplier delays and production delays more rapidly, and improve decisions with the new data (or for new suppliers or new customers).”
Gartner, 2021
Importantly, Gartner does not believe that a data fabric is something that can be built in its entirety, or likewise bought off-the-shelf as a complete solution. In fact, it is quite adamant that data and analytics leaders should be pursuing an approach that pairs best-of-breed solutions commercially available in the market with the firm’s own in-house solutions.
“No existing stand-alone solution can facilitate a full-fledged data fabric architecture. D&A leaders can ensure a formidable data fabric architecture using a blend of built and bought solutions. For example, they can opt for a promising data management platform with 65-70% of the capabilities needed to stitch together a data fabric. The missing capabilities can be achieved with a homegrown solution.”
Gartner, 2021
Besides Gartner, other industry experts have written on the differences between data fabric and data mesh as being primarily about how data is accessed, and by whom. James Serra of EY has said that data fabrics are technology-centric, but data meshes are targeting organisational change.
A data fabric might therefore overlay atop various data repositories, and bring some unification in management of the data. It can then provide downstream consumers of the data – stewards, engineers, scientists, analysts and senior management – with meaningful intelligence.
Data meshes however are more about empowering groups of teams to manage data as they see fit in line with a common governance policy. At the moment, lots of companies employ Extract, Transform and Load (ETL) pipelines to try and keep data aligned, and consistent. Data meshes advocate the concept of “data as a product” – rather than simply a common governance policy, data can be shaped into products for use by the business.
The Datactics view on the benefits of a Data Fabric approach
In our experience, there are a wide range of business benefits to adopting a data fabric. Generally, organisations can benefit from a unified data approach as it fundamentally simplifies access to enterprise data and reduces the amount of data silos. Having distributed data across an organisation can hinder efficient operations, but by making data accessible to stewards and data engineers across the organisation, businesses can benefit from greater interoperability and as a result, make better decisions.
In the context of data quality specifically, a data fabric implementation provides the optimum architecture to apply data quality controls to a large volume of critical data assets, helping you achieve a more unified view of your data quality. Monitoring data in transit (compared to data at rest) helps to react more quickly to data quality issues and is a step towards a more proactive data quality approach.
However, a data fabric can create an enterprise-wide demand for uniformity of technologies, which may or may not suit the business needs or business model.
The Datactics view on the benefits of a Data Mesh approach
Because data meshes prioritise organisational change over the adoption of more technology, a data mesh is an approach that is typically favoured by organisations that are not intent on pursuing top-down governance over bottom-up agile working methodologies. It doesn’t always mean that no new technology will be required to design and deploy a data mesh, because each function will have to be able to create and deliver data-as-a-product to an agreed level of quality and in compliance with internal and external standards. Additionally, a data mesh will suit teams who do not have their own coders, and instead rely on business and subject matter expertise allied to no-code tools for a wide range of data management and data quality operations.
In this case, there is less call for technology uniformity, and more freedom for distributed teams to build systems that meet their own needs, albeit with cross-team and cross-function governance provisions.
Data Fabric and Integration
Gartner explains that a robust data fabric must facilitate traditional methods of data integration, such as processing data and ETL. It also must be capable of supporting all users, from data stewards to business users wanting to self-serve in their data analytics
Similarly, by leveraging machine learning, a data fabric monitors existing data pipelines and analyses metadata in order to connect multiple data sources from across an organisation. This makes it much easier for a data scientist to interpret the information and improve data analytics.
By its very nature, a data fabric needs to support integration and this is where the Datactics data quality solution can add value when building a data fabric framework.
Data Mesh and Integration
There’s less of a priority on data integration for data meshes, however interoperability of the distributed data management environments is an absolute must. If components of a data management platform do not interoperate, or have no API connectivity (for example), then it is going to be time to explore alternatives that do!
How the Datactics solution complements Data Fabrics and Data Meshes
As highlighted in this year’s Gartner Magic Quadrant, Datactics is a ‘best of breed’ Data Quality tool – we do Data Quality exceptionally well (ask our clients!). However, Datactics recognizes the fact that Data Quality is only one piece of the overall data management puzzle and data integration is a key component in our delivery process.
In order to help our clients build a data fabric architecture, we must connect easily with other tools. Being able to integrate with other areas of the data management ecosystem is something Datactics does well. Our solution integrates seamlessly with solutions ranging from Data Governance to Data Lineage and Master Data Management.
Integration is fundamental to the design of our platform, which offers frictionless connectivity to other vendor tools via API and other means. We don’t plan on adding data catalogue or data lineage capabilities to the Datactics platform. However, we will connect with existing ‘best in breed’ tools using an open metadata model. This therefore creates an integrated system of best of breed data management capabilities.
Datactics are no strangers when it comes to connecting with a variety of data sources and systems. The very nature of Data Quality means that Datactics needs to connect to data from across a client’s entire estate- including cloud platforms, data lakes, data warehouses, business applications and legacy systems. Connecting to these data sources and systems needs to be robust in order to perform data quality measurement and remediation processes.
How does Datactics approach integration with specialist data management tools?
When developing or enhancing its data management programme, we appreciate that an organisation will want to integrate a new solution seamlessly with (potentially) multiple other data systems and vendors. This is helped by the abundance of connectivity options available in the Datactics platform, to integrate with existing systems and vendors in order to make it easier for businesses to establish a sustainable Data Fabric.
A good example of where integration can add real business value is through the combination of Data Quality and Data Lineage. The automated technical lineage information provided by Manta provides Datactics with the ‘coordinates’ to point Data Quality rules to a larger volume of critical data elements within a data set. As a result, data quality is more effectively rolled out across an organisation.
Similarly, as Datactics measures data quality in-motion across multiple source systems & business applications, DQ metrics can be visually represented in the excellent metadata model visualisation provided by Solidatus. This allows users to identify the root cause of a data quality issue very quickly and trace the downstream impacts on a client’s business processes.
Another natural area of integration is between Data Quality and Data Governance systems. Data ownership metadata & data quality rules definitions housed in these systems can be pulled into Datactics via REST API. Meanwhile, metadata on the rules input and data quality metrics on the data assets can be pushed back into the Governance or Catalog system.
Other systems Datactics connects with are Business Intelligence and visualisation tools, ticketing systems and Master Data Management systems. For instance, the software ships with out-of-the-box connectivity to off-the-shelf tooling such as Qlik, Tableau, and PowerBI on the visualisation side, and Jira and Service Now on the ticketing front.
Next steps
If you are developing a data management framework, exploring data fabric or data mesh architecture. or are simply seeking to understand open integration of best-of-breed data quality technologies and would like to hear more about our integration capabilities, please reach out to Kieran Seaward or contact us.